Chapter 15. Mixed Methods
Introduction
Where deep ethnography (chapter 14) is a tradition that relies on naturalistic techniques of data collection, foregrounding the specificity of a particular culture and site, there are other times when researchers are looking for approaches that allow them to make use of some of the analytical techniques developed by statisticians and quantitative researchers to generalize the data they are collecting. Rather than push into a deeper understanding of a culture through thick interpretive descriptions, these researchers would rather abstract from a sufficiently large body of cases (or persons) to hazard predictions about a connection, relationship, or phenomenon. You may already have some experience learning basic statistical techniques for analyzing large data sets. In this chapter, we describe how some research harnesses those techniques to supplement or augment qualitative research, mixing methods for the purpose of building stronger claims and arguments. There are many ways this can be done, but perhaps the most common mixed methods research design involves the use of survey data (analyzed statistically via descriptive cross-tabs or fairly simple regression analyses of large number probability samples) plus semistructured interviews. This chapter will take a closer look at mixed methods approaches, explain why you might want to consider them (or not), and provide some guidance for successful mixed methods research designs.
What Is It? Triangulation, Multiple Methods, and Mixed Methods
First, a bit of nomenclature. Mixed methods can be understood as a path toward triangulation. Triangulation is a way of strengthening the validity of a study by employing multiple forms of data, multiple investigators, multiple theoretical perspectives, or multiple research methods. Let’s say that Anikit wants to know more about how first-year college students acclimate to college. He could talk to some college students (conduct interviews) and also observe their behavior (fieldwork). He is strengthening the validity of his study by including multiple forms of data. If both the interview and the observations indicate heavy reliance on peer networks, a reported finding about the importance of peers would be more credible than had he only interviewed students or only observed them. If he discovers that students say one thing but do another (which is pretty common, after all), then this, too, becomes an interesting finding (e.g., Why do they forget to talk about their peers when peers have so much observable influence?). In this case, we say that Anikit is employing multiple forms of data, or even that he relies on multiple methods. But he is not, strictly speaking, mixing data. Mixed methods refer specifically to the use of both quantitative and qualitative research methods. If Anikit were to supplement his interviews and/or observations with a random sample of one thousand college students, he would then be employing a mixed methods approach. Although he might not get the rich details of how friends matter in the survey, the large sample size allows statistical analyses of relationships among variables, perhaps showing which groups of students are more likely to benefit from strong peer networks. So to summarize, both multiple methods and mixed methods are forms of research triangulation,[1] but mixed methods include mixing both qualitative and quantitative research elements.
Mixed methods techniques, then, are pretty unique. Where many qualitative researchers have little interest in statistical generalizability, and many quantitative researchers undervalue the importance of rich descriptions of singular cases, the mixed methods researcher has an open mind about both approaches simultaneously. And they use the power of both approaches to build stronger results:[2]
Quantitative (mainly deductive) methods are ideal for measuring pervasiveness of “known” phenomena and central patterns of association, including inferences of causality. Qualitative (mainly inductive) methods allow for identification of previously unknown processes, explanations of why and how phenomena occur, and the range of their effects (Pasick et al. 2009). Mixed methods research, then, is more than simply collecting qualitative data from interviews, or collecting multiple forms of qualitative evidence (e.g., observations and interviews) or multiple types of quantitative evidence (e.g., surveys and diagnostic tests). It involves the intentional collection of both quantitative and qualitative data and the combination of the strengths of each to answer research questions. (Creswell et al. 2011:5; emphases added)
Why Use Mixed Methods?
As with all methodological choices, the answer depends on your underlying research questions and goals. Some research questions are better answered by the strengths of the mixed methods approach. Small (2011) discusses the use of mixed methods as a confirmation or complement of one set of findings from one method by another. Creswell and Clark (2017:8ff.) note the following situations as being particularly aided by combining qualitative and quantitative data collection and analysis: (1) when you need to obtain both more complete (need for qualitative) and more corroborated (need for quantitative) information; (2) when you need to explain (need for qualitative) initial results (quantitative); (3) when you need to do an exploratory study (need for qualitative) before you can really create and administer a survey or other instrument (quantitative); (4) when you need to describe and compare different types of cases to get a more holistic understanding of what is going on; (5) when you need (or very much want!) to include participants in the study, adding in qualitative elements as you build a quantitative design; (6) when you need all the tools at your disposal to develop, implement, and evaluate a program.
Please note what is not included in this list: because you can. Mixed methods research is not always preferable, even if in general it makes your study “stronger.” Strength is not the only criterion for quality or value. I have met many students in my career who assume that the mixed methods approach is optimal because it includes both qualitative and quantitative research. That is the wrong way of looking at things. Mixed methods are optimal when and only when they fit the necessities of your research question (e.g., How can I corroborate this interesting finding from my interviews so that proper solutions can be fashioned?) or underlying goal (e.g., How can I make sure to include the people in this program as participants of the study?).
If you are just starting out and learning your way through designing your first study, mixed methods are not default requirements. As you will see in the next section on design, mixed methods studies often happen sequentially rather than consecutively, so I recommend you start with the study that has the most meaning to you, the one that is the most compelling. Later on, if you want to add (mix) another approach for the sake of strength or validity or “corroboration” (if you are adding quantitative) or “explanation” (if you are adding qualitative), you can always do that then, after the completion of your first study.
Segue: Historical Interlude
For those interested in a little history, one could make the case that mixed methods research in the social sciences actually predates the development of either quantitative or qualitative research methods. The very first social scientists (what we call “social science” in the West, which is itself a historical construct, as many other peoples have been exploring meaning and interpretation of the social world for centuries if not millennia) often employed a mélange of methods to address their research questions. For example, the first sociologists in the US operating out of the “Chicago School” of the early twentieth century surveyed neighborhoods, interviewing people, observing demographic subcultures, and making tallies of everything from the numbers of persons in households to what languages were being spoken. They learned many of these techniques from early statisticians and demographers in Europe—people like Charles Booth (1902), who surveyed neighborhoods in London, and Frédéric Le Play, who spent decades examining the material conditions of the working classes across Europe, famously including family “budgets” along with interviews and observations (see C. B. Silver 1982). The renowned American sociologist W. E. B. Du Bois, who was the first Black man to earn a PhD from Harvard University, also conducted one of the very first mixed methods studies in the US, The Philadelphia Negro (1899). This work mapped every Black residence, church, and business in Philadelphia’s Seventh Ward and included observations and details on family structure and occupation (similar to Booth’s earlier work on London). Continuing through the 1930s and 1940s, “community studies” were conducted by teams of researchers who basically tallied everything they could find about the particular town or city they chose to work in and performed countless interviews, months and years of fieldwork, and detailed mappings of community relationships and power relations. One of the most famous of these studies includes the “Middletown” studies conducted by Robert and Helen Lynd (1929, 1937).
As statistical analysis progressed after World War II alongside the development of the technology that allowed for ever faster computations, quantitative research emerged as a separate field. There was a lot to learn about how to conduct statistical analyses, and there were more refinements in the creation of large survey instruments. Qualitative research—the observations and interviews at the heart of naturalistic inquiry—became a separate field for different kinds of researchers. One might even say qualitative research languished at the expense of new developments of quantitative analytical techniques until the 1970s, when feminist critiques of positivist social science emerged, casting doubt on the superiority of quantitative research methods. The rise of interdisciplinarity in recent decades combined with a lessening of the former harsh critique of quantitative research methods and the “paradigm wars” (Small 2011) has allowed for an efflorescence of mixed methods research, which is where we are today.
Mixed-Methods Research Designs
Returning from our historical interlude to the list of possible uses of mixed methods, we now confront the question of research design. If we are using more than one method, how exactly do we do this, and when? The how and the when will depend largely on why we are using mixed methods. For example, if we want to corroborate findings emerging from interviews, then we obviously begin with interviews and follow with, perhaps, a large survey. On the other hand, if we are seeking to explain findings generated from a survey, we begin with that survey and add interviews or observations or focus groups after its completion. And if we are seeking to include participants in the research design itself, we may want to work concurrently, interviewing and holding focus groups as surveys are administered. So it all depends on why we have chosen to use mixed methods.
We can think of our choices here in terms of three possibilities. The first, called sequential explanatory, begins with quantitative data (collection) and then follows with qualitative data (collection). After both are collected, interpretations are made. The second, called sequential exploratory, begins the other way around, with qualitative followed by quantitative. After both are collected, interpretations are made. The third, called concurrent triangulation, conceives of both quantitative and qualitative elements happening concurrently. In practice, one may still happen before the other, but one does not follow the other. The data then converge, and from that convergence, interpretations are made.
In sequential explanatory design (figure 15.1), we are asking ourselves, “In what ways do the qualitative findings explain the quantitative results?” (Creswell et al. 2017). This design thus gives some priority to the quantitative data. The qualitative data, collected after the quantitative data, is used to provide a better understanding of the research problem and then the quantitative data alone.
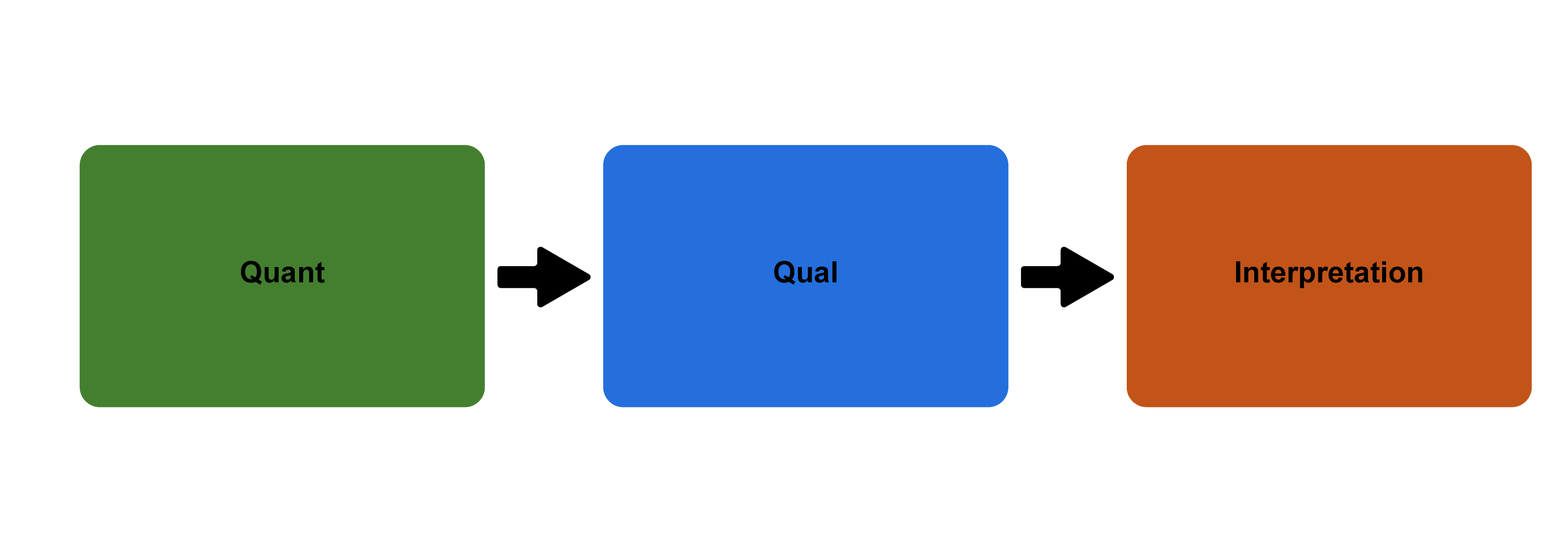
Often, this means providing some context or explaining meanings and motivations behind the correlations found in the quantitative data. For example, in my research on college students (Hurst 2019), I found a statistical correlation between upper-middle-class female students and study abroad. In other words, and stating this rather baldly, class*gender could be used to predict who studied abroad. But I couldn’t fully explain why, given the survey data I had collected.[3] To answer these (and other) questions that the survey results raised, I began interviewing students and holding focus groups. And it was through these qualitative forms of data collection that I found a partial answer: upper-middle-class female students had been taught to see study abroad as a final “finishing” component of their education in a way that other students simply had not. They often had mothers who had done the same. And they clearly saw connections here to the kinds of well-traveled cosmopolitan adults they wanted to become.
In sequential exploratory design (figure 15.2), we are asking ourselves, “In what ways do the quantitative findings generalize (or confirm) the qualitative results?” (Creswell et al. 2018). This design thus gives some priority to the qualitative data. The quantitative data, collected after the qualitative data, is used to confirm the findings.
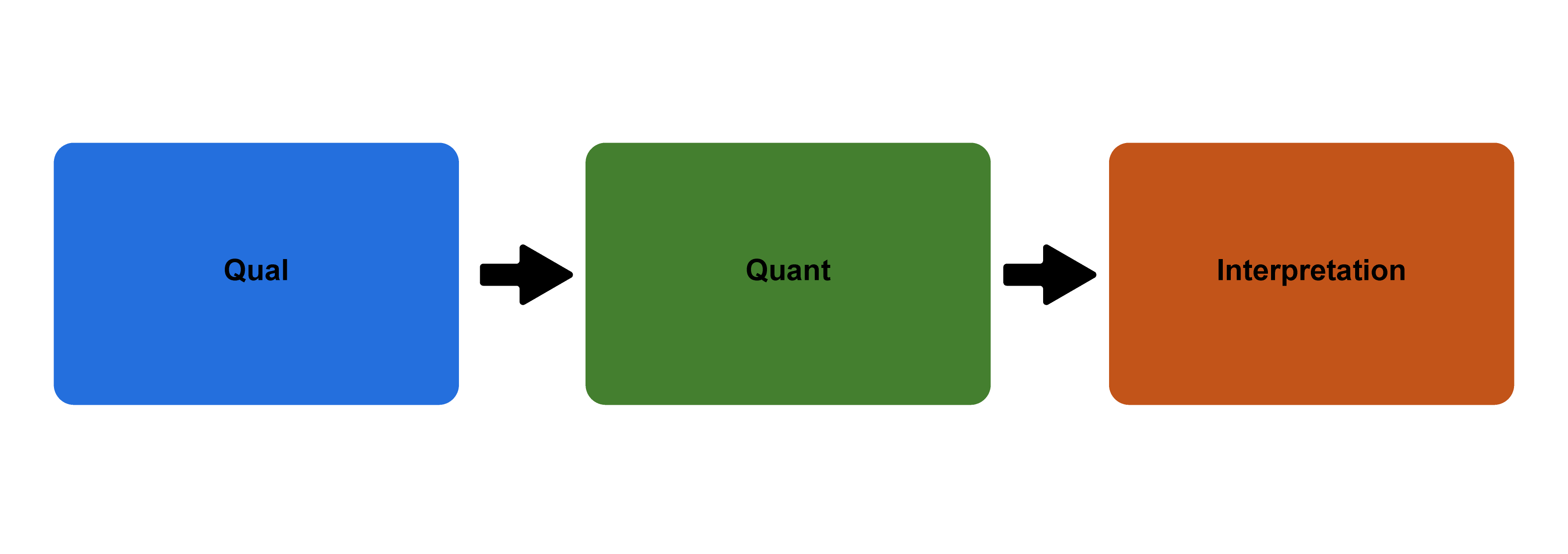
This approach is ideal for developing new instruments or when a researcher intends to generalize findings from a qualitative study to different groups or populations. The American Sociological Association (ASA) Task Force on First-Generation and Working-Class Persons wanted to understand how class background may have played a role in the success of sociology graduate students and faculty. Because this was a relatively new research question, the task force began by conducting several focus groups, asking general questions about how class might have affected careers in sociology. Based on several recurring findings (e.g., high debt burdens, mentorship, feelings of fit), the task force developed a survey instrument that it then administered to more than one thousand sociologists, thus generalizing the preliminary findings and providing corroboration of some of the key variables at play.
In concurrent triangulation design (figure 15.3), neither the quantitative nor the qualitative component takes precedence. Although in practice one might precede the other in time, neither is the tail that wags the dog, so to speak. They are both the dog. The general of this design is to better understand or deepen one’s understanding of the phenomenon under study. The goal is to obtain different but complementary data that strengthen (validate) the overall results.
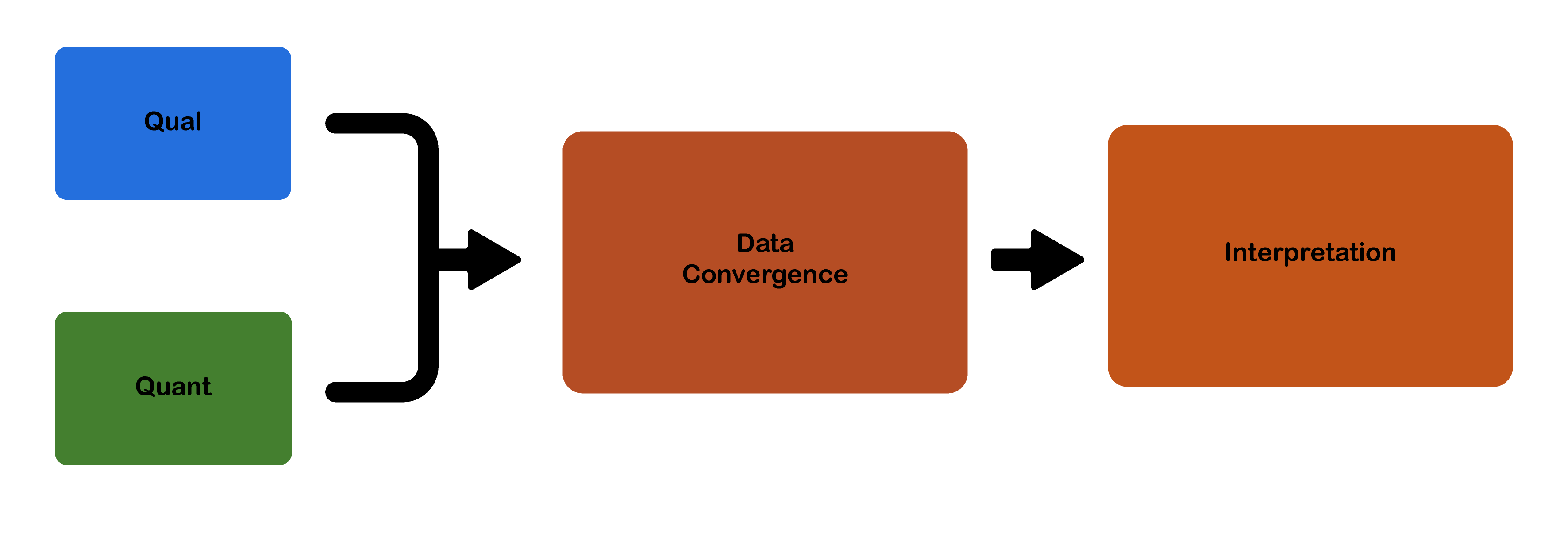
These designs might be either nested or nonnested. In a nested design, a subsample of an original randomized sample is used for further interviews or observation. A common nested design form is where in-depth interviews are conducted with a subsample of those who filled out a survey. Nonnested designs occur when it is impractical or impossible to recruit the same individuals that took place in the survey. The research I conducted for my book Amplified Advantage (Hurst 2019) is an example of this. I supplemented a large national survey of college students and recent college graduates with interviews and focus groups of similar college students and graduates who were not participants in the study (or who may have been randomly selected as participants but without my knowledge or linking their data). Nonnested designs are much more flexible than nested designs, but they eliminate the possibility of linking data across methods.
As with all research design, it is important to think about how best to address your particular research question. There are strengths and weaknesses of each design. Sequential design allows for the collection and analysis of different methods separately, which can make the process more manageable. Sequential designs are relatively easy to implement, design, and report. Sequential exploratory designs allow you to contextualize and generalize qualitative findings to larger samples, while sequential explanatory designs enable you to gain a deeper understanding of findings revealed by quantitative data analysis. All sequential design takes a lot of time, however. You are essentially doubling your research. This is why I do not recommend these approaches to undergraduate students or graduate students in master’s programs. In contrast, concurrent designs, whose dual methods may be conducted simultaneously, may be conducted more quickly. However, as a practical matter, you will probably end up focusing first on one data collection method and then the other, so the time saved might be minimal.[4] Concurrent design can also preclude following up on interesting findings that emerge from one side of the study, and the abbreviated form may prevent clarification of confusing issues that arise during analysis. If the results are contradictory or diverge, it may also be difficult to integrate the data. You might end up with more questions to pursue for further study and not much conclusive to say at the end of all your work.
Finally, there is what I will call here the recursive design model (figure 15.4), in which you combine both explanatory and exploratory sequential design.
This design is currently being used by the ASA task force mentioned above. The first stage of data collection involved several focus groups. From these focus groups, we constructed a survey that we administered to ASA members. The focus group survey could be viewed as an example of exploratory sequential design. As the surveys were being analyzed, we added a nested set of interviews with persons who had taken the survey and who indicated a willingness to participate in this later stage of data collection. These interviews then help explain some of the findings from the survey. The entire process takes several years, however, and involves multiple researchers!
Advanced: Crossover Design
Small’s (2011) review of the state of mixed methods research argues that mixed methods are being increasingly adopted in social science research. In addition to sequential and concurrent research designs, where quantitative and qualitative data work to either confirm or complement each other, he sets forth examples of innovative designs that go further toward truly blending the special techniques and strengths of both quantitative and qualitative methods.[5] Written in 2011, I have seen scant evidence so far that these blended techniques are becoming well established, but they are promising. As new software programs for data analysis emerge, along with increased computing power, there will be greater opportunities for crossover work. Perhaps you can take up the charge and attempt one of these more innovative approaches yourself.
Here is Small’s (2011:73ff.) list of innovative crossover research design:
- Network analyses of narrative textual data. Here, researchers use techniques of network analysis (typically quantitative) and apply them to narratives (qualitative), coding stories as separate “nodes” and then looking for connections between those nodes, as is done in network analysis.
- Sequence analyses of narrative textual data. Here, techniques of event structure analysis and optimal matching (designed for analysis of quantitative data) are applied to narratives (qualitative data). The narratives are reconceived as a series of events, and then causal pathways between these events are mapped. This allows for identification of crucial turning points as well as “nonsignificant” events that just happened.
- Quantitative analyses of semantic (meaning) elements of narrative textual data. The basic distinction between quantitative (data in the form of numbers) and qualitative (date in the form of words) gets blurred here, as words themselves and their meanings and contexts are coded numerically. I usually strongly advise beginning students to do this, as what often happens is that they begin to think quantitatively about the data, flattening it considerably. However, if done with full attention to meaning and context, the power of computing/analytical software may strengthen the coding process.
- Narrative analyses of large-n survey data. In contrast to the first three designs listed above, where quantitative techniques were applied to qualitative data, we now come to a situation where the reverse takes place. Here we have a large data set, either coded numerically or “raw” with various choice options for each question posed. Rather than read the data set as a series of factors (variables) whose relationship one explores through statistical analyses, the researcher creates a narrative from the survey responses, contextualizing the answers rather than abstracting them.[6]
- Regression-based analyses of small-n or narrative textual data. This is by far the most common crossover method and the reverse of the fourth example. Many qualitative software analysis programs now include basic quantitative analytical functions. The researcher can code interview transcripts and fieldnotes in such a way that allows for basic cross-tabulations, simple frequency statistics, or even basic regression analyses. Transcripts and fieldnotes can generate “variables” for such analyses.
Despite the promise of blending methods in this way, the possibility of doing damage to one’s study by discounting the particular values of either quantitative or qualitative approaches is a real one. Unlike mixed methods, where the two approaches work separately (even when designed to concur in time), crossover research blends or muddies the two. Small (2011) warns, “At a minimum, the application of techniques should not be fundamentally contrary to the epistemological principles from which they are derived or to the technical problems for which they were intended” (76). When employing any of these designs or blending approaches, it is very important to explain clearly and fully what one’s aims are and how the analysis has proceeded, as this allows others to evaluate the appropriateness of the design for the questions posed.
Further Readings
Cech, Erin. 2021. The Trouble with Passion: How Searching for Fulfillment at Work Fosters Inequality. Berkeley, CA: University of California Press.* Cech combines surveys with interviews to explore how people think about and talk about job searches and careers.
Cooper, Kristy S. 2014. “Eliciting Engagement in the High School Classroom: A Mixed-Methods Examination of Teaching Practices.” American Educational Research Journal 51(2):363–402. An example of using multilevel regression analyses with both interviews and observations to ascertain how best to engage students.
Creswell, John W., and J. David Creswell. 2018. Research Design: Qualitative, Quantitative, and Mixed Methods Approaches. Thousand Oaks, CA: SAGE. Essential textbook for mixed-methods research.
Edin, Kathryn, and Maureen A. Pirog. 2014. “Special Symposium on Qualitative and Mixed-Methods for Policy Analysis.” Journal of Policy Analysis and Management 33(2):345–349. A good overview of the strengths of mixed-methods research, which, the authors argue, make it particularly well suited for public policy analysis.
Hurst, Allison L. 2019. Amplified Advantage: Going to a “Good” College in an Era of Inequality. Lanham, MD: Rowman & Littlefield: Lexington Books..* Employs a national survey of recent graduates of small liberal arts colleges combined with interviews, focus groups, and archival data to explore how class background affects college outcomes.
Johnson, R. Burke, and Anthony J. Onwuegbuzie. 2004. “Mixed Methods Research: A Research Paradigm Whose Time Has Come.” Educational Researcher 33(7):14–26. Takes a pragmatic approach and provides a framework for designing and conducting mixed-methods research.
Klinenberg, Eric. 2015. Heat Wave: A Social Autopsy of Disaster in Chicago. Chicago: University of Chicago Press.* A great read and could not be more timely. Klinenberg uses a combination of fieldwork, interviews, and archival research to investigate why some neighborhoods experience greater mortality than others.
Lynd, Robert, and Helen Lynd. 1929. Middletown: A Study in American Culture. New York: Harcourt, Brace.* This early mixed-methods study of a “typical” American city was a pioneering work in sociology. The husband-and-wife team seemingly explores every aspect of life in the city, mapping social networks, surveying attitudes and beliefs, talking to people about their expectations and lives, and observing people going about their everyday business. Although none of the techniques are very sophisticated, this remains a classic example of pragmatic research.
Lynd, Robert, and Helen Lynd. 1937. Middletown in Transition. New York: Harcourt, Brace. The follow-up to the Lynds’ original study of a small American city. More theoretical and critical than the first volume.
Markle, Gail. 2017. “Factors Influencing Achievement in Undergraduate Social Science Research Methods Courses: A Mixed Methods Analysis.” Teaching Sociology 45(2):105–115.* Examines the factors that influence student achievement using an initial survey with follow-up interviews.
Matthews, Wendy K. 2017. “‘Stand by Me’: A Mixed Methods Study of a Collegiate Marching Band Members’ Intragroup Beliefs throughout a Performance Season.” Journal of Research in Music Education 65(2):179–202.* The primary method here is focus groups, but the author also employed multivariate analysis of variance (MANOVA) to shore up the qualitative findings.
Monrad, Merete. 2013. “On a Scale of One to Five, Who Are You? Mixed Methods in Identity Research.” Acta Sociologica 56(4):347–360. A call to employ mixed methods in identity research.
Silver, Catherine Bodard. 1982. Frédéric Le Play on Family, Work and Social Change. Chicago: University of Chicago Press. For anyone interested in the historic roots of mixed-methods research, the work of Frédéric Le Play is essential. This biography is a good place to start.
Small, Mario Luis. 2011. “How to Conduct a Mixed Methods Study: Recent Trends in a Rapidly Growing Literature.” Annual Review of Sociology 37:57–86. A massive review of recent mixed-methods research, distinguishing between mixed-data-collection studies, which combine two or more kinds of data, and mixed-data-analysis studies, which combine two or more analytical strategies. Essential reading for graduate students wanting to use mixed methods.
- To extend this notion of triangulation a little further: if Anikit enlisted the help of Kanchan to interpret the observations and interview transcripts, he would be strengthening the validity of the study through multiple investigators, another form of triangulation having nothing at all to do with what methods are employed. He could also bring in multiple theoretical frameworks—say, Critical Race Theory and Bourdieusian field analysis—as a form of theoretical triangulation. ↵
- If stronger is your aim, that is. For many qualitative researchers, verisimilitude, or the truthfulness of a presentation, is a more desirable aim than strength in the sense of validity. ↵
- Actually, I could do a fair amount of testing on other variables’ relationships to this finding: students who had gone far away to college (more than five hundred miles) were significantly more likely to study abroad, for example, as were students who majored in arts and humanities courses. But I still missed any way of getting at personal motivations or how individuals explained these motivations. That is the part a survey is just never going to fully get at, no matter how well or numerous the questions asked. ↵
- The big exception here is when you are relying on data that has already been collected and is ready for analysis, as in the case of large survey data sets like the General Social Survey. In that case, it is not too time consuming to design a mixed methods study that uses (nonnested) interviews to supplement your analyses of survey data. ↵
- I refer to these as blended methods rather than mixed methods because the epistemological positions and science claims, usually rather distinct from quantitative (more positivistic) and qualitative (more naturalistic), blur considerably. ↵
- I admit that trained first as a qualitative researcher, this has always been my impulse when confronting a large survey data set. ↵
A research design that employs both quantitative and qualitative methods, as in the case of a survey supplemented by interviews.
The process of strengthening a study by employing multiple methods (most often, used in combining various qualitative methods of data collection and analysis). This is sometimes referred to as data triangulation or methodological triangulation (in contrast to investigator triangulation or theory triangulation). Contrast mixed methods.
A mixed-methods design that conceives of both quantitative and qualitative elements happening concurrently. In practice, one may still happen before the other, but one does not follow the other. The data then converge and from that convergence interpretations are made. Compare sequential exploratory design and sequential explanatory design.
A mixed-methods design that begins with quantitative data collection followed by qualitative data collection, which helps “explain” the initial quantitative findings. Compare sequential exploratory design and concurrent triangulation.
A mixed-methods design that begins with qualitative data collection followed by quantitative data collection. In this case, the qualitative data suggests factors and variables to include in the quantitative design. Compare sequential explanatory design and concurrent triangulation.
A form of mixed-methods design in which a subsample of an original randomized sample is used for further interviews or observation.