Section 4 – Methods
You should not be universally skeptical, but also not universally trusting. You need to understand how and why certain methods work and others do not. Aligning methods and questions may be the most important skill for the future. In this section I will layout a number of methods for doing research in media, with the hope that you will understand what good research looks like in each area, so that you may ignore and refute the bad.
Methodology is a loop. Without qualities, there are no meaningful qualities. Without some sense of quantity, qualities are unmoored from reality. There is no qualitative answer that can eliminate the need to count, just as there is no way to count to an assembled claim. Communication Studies is a lucky discipline as our questions are not welded to a single methodological chassis.
4.1 Qualitative
Qualitative research presents descriptions, interpretations, and criticism. The question of description: what was the text? What does it mean? How do the codes work? To what end were those codes selected?
Qualitative research is primarily concerned with the qualities of the cases in the study in question. Computational methods tend to impart indolence with regard to the question of the data themselves or worse an aggressive pruning of ambiguity which makes for clean data, but poor models of human communication. Great qualitative research is rigorous, deeply informed, and essential to decision making, ethics, and meaning.
4.1.1 Ethnography
As a practice of tracing the everyday performance of individuals, ethnographic research, particularly field work, sees the body and experience as the basis for analysis. Researchers in this tradition conduct interviews and spend time in the field. Rigor comes from demonstrating that one was really embedded in the system of meanings that are used by a group of people or in a particular context. To maintain a structured system of thinking, the researcher continuously produces field notes, which serve as an intermediate document of what things meant to the individual at a particular point in time. These structured notes then allow the researcher to remember key details to construct the finished account later. Finalized research then is the product of a review of these notes and a reconstruction of the symbolic system. This research is not published as a sort of abstract formula, but as a rich account of the meaning itself. Great ethnographic work may read more like a novel than a lab report.
Dwight Conquergood, a major ethnographer of communication, in his development of a critical ethnographic practice called for the ongoing revaluation of the sources of authority for those who would claim to know what meaning is in a culture. The key to this performance ethnography is constant self-reflexity: the researcher needs to understand how their mode of presenting themselves and the world produces their own academic authority. Descriptions of hardship often seem to provide ethnographers credibility. Why such an intense struggle? Meaning is owned by a group, it is not hard to find many troubling examples of cultural appropriation where information is scooped up and resold as a product with little remuneration of the original producers. More instrumentally, there could be multiple explanations of the same phenomena. Ethnographers have many interesting strategies for demonstrating rigor and building credibility. When these are well deployed, and when the account is ethical and engages the community, the results are profound. It makes sense why large corporations like Microsoft pay for academic ethnographic research. These are the insights that can make a game changing product.
It is the power of reflexive ethnography that is often mistaken as the seemingly magical dimension of design. If the designer is truly, reflexively integrated into the use context of a thing or idea their designs will really resonate in a powerful way. At the same time, it becomes comically excessive when people who are not embedded claim the same sort of authority or to make the same claims to meaning as those who have not engaged. But wait – notice that in the creation of authority for the ethnographer in the last sentence I have already deployed a rhetoric of effort and time, as if that work somehow gives a person the right to make authoritative claims about meaning simply on the basis of duration. Would it make the claim more powerful if I said they experienced hardship?
Ethnography is a powerful approach, and people who have the patience and the reflexive sense to do this well are rare. At the same time, this is not a special property of some people, but a skill that is refined over many years. It is entirely possible to do meaningful work in this area that does not rise to the level of publishable ethnographic research. Interview and observation methods have real value, and can contribute to your efforts. Good research in this vein will be deliberate and reflective, making modest claims with abundant evidence. Bad research will be quick and easy, a cover for pre-established conclusions and bad ideas.
4.1.2 Hermeneutics
The hermeneutic tradition comes from an ancient sort of grandmother discipline called philology which focused on textual interpretation. Many of the discussions of semiotics and particular media in this book offer approaches to understanding meaning.
The traditional seven question typology of hermeneutics as presented in the Stanford Encyclopedia of Philosophy:
- Who (is the author) (quis/persona)?
- What (is the subject matter of the text) (quid/materia)?
- Why (was the text written) (cur/causa)?
- How (was the text composed) (quomodo/modus)?
- When (was the text written or published) (quando/tempus)?
- Where (was the text written or published) (ubi/loco)?
- By which means (was the text written or published) (quibus faculatibus/facultas)?[1]
I often recommend these as a starting point for any student looking to understand their readings in class. These questions can help you reconstruct the state of an academic discipline or in the original case, to reconstruct the text of the Bible. Many of the ideas in hermeneutics are similar to those deployed in the theorization of the conditions of possibility in communication studies.
Hermeneutic approaches follow a tradition called close reading, this employs an interpretive strategy to be used on a particular text. Good work in this area will employ a structured framework and demonstrate how different parts of the code work. Ultimately it is about the code. Or as Jacques Derrida put it: there is no outside of the text.[2] A deep account of who wrote what, when, where and why can change how you think.
For undergraduate audiences, hermeneutic critique is presented as the work of the “masters of suspicion” (Nietzsche, Marx, Freud) as designated by Paul Ricoeur – this presentation flattens a number of unique interesting questions presented by each author, and tends to position the world in a troubling critical/non-critical binary.[3] In her critique of this binary, Rita Felski argues that the binary misses the range of possibilities and texture that come from any number of approaches to interpreting the text:
In a related essay, I scrutinize some of the qualities of a suspicious or critical reading practice: distance rather than closeness; guardedness rather than openness; aggression rather than submission; superiority rather than reverence; attentiveness rather than distraction; exposure rather than tact (215–34). Suspicion, in this sense, constitutes a muted affective state—a curiously non-emotional emotion of morally inflected mistrust—that overlaps with, and builds upon, the stance of detachment that characterizes the stance of the professional or expert. That this style of reading proves so alluring has much to do with the gratifications and satisfactions that it offers. Beyond the usual political or philosophical justifications of critique, it also promises the engrossing pleasure of a game-like sparring with the text in which critics deploy inventive skills and innovative strategies to test their wits, best their opponents, and become sharper, shrewder, and more sophisticated players.[4]
Skilled critics working with codes can do great work. We should take Felski’s warning seriously as we develop new interpretative frames for the future but also to understand when we should engage in the practice of translational critique. This is an important political moment in any academic project: when is the theory complete and the time for intervention?
4.1.3 Historicism
In 2015, a film crew went on a dingy quest in New Mexico in search of lost video games, in this case 881 ET Atari cartridges.[5] The question is why? The cartridges were dumped because of the lack of meaningful control of the production of games for the 2600 which resulted in a glut of terrible games. For undergraduate teaching this is a wonderful example – you can teach the political economy of the game industry, touch on the legal structures which enable production, and teach key points in history of the product: all at the same time.
Media history is a nexus that allows the introduction of compelling questions. Much of the excellent work of John Durham Peters has been concerned with the development of logistical media technologies (like calendars and towers).[6] The inspiration of Frierich Kittler on Peters should be apparent, with an important caveat: Peters tends to assume that the driving force for media development is longing, Kittler assumes armed conflict.[7] There are any number of ways of thinking and assembling evidence, for media historians the work is always translational, the assembly of ideas and archives are intended to work along an explanatory line. For more traditional academic historians this may be premature, as the inductive collection of facts in bulk is necessary before embarking on a structured pattern of noticing.
Good historical work actively reduces its own scope. Historical research expands geometrically – every time the project attempts to explain something else it grows to include the new idea and all the contact points between the idea and the existing project.
History, when done well, provides the most detailed possible accounting for the conditions of possibility for the present.
One of the most common forms of historical reason that you will see is not the academic history, but the business case study. A case is isolated in time and reduced to what Wes Rumelt describes as a kernel, where a single set of coherent policies could have produced actions that would have changed the direction of a business enterprise.[8] Business reasoning, as a form of historicism, assumes that the understanding of past forces can then be inferred as a continuous function that will shape the future. The greatest flaw to this sort of media history is that the accounts created will almost always feature “winners” discussing their brilliance. Narrow, self-serving, yet historical. Both undergraduates and MBA students will be writing cases for the foreseeable future. It should be clear that this mode of reasoning is in common use in everyday life and it can help us understand why it would be a good use of time and money to dig up a landfill.
4.1.4 Rhetoric
Rhetoric is an academic field that emphasizes the probability of effect for a particular utterance especially if that is a persuasive utterance. This field deeply informs this book and the impact of it can be seen across the text.
4.1.5 Policy
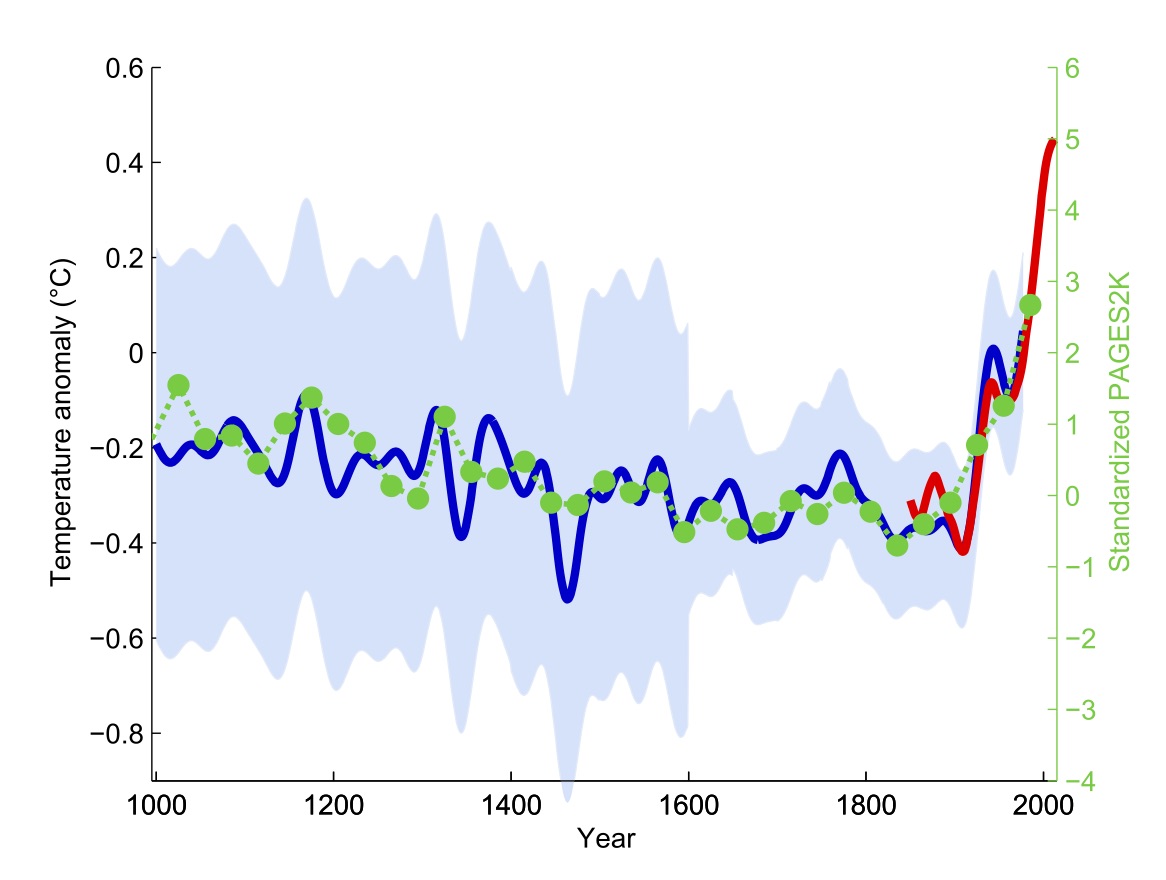
Policy analysis is concerned with the translation of other findings about the world into a meaningful course of action. This is normative, meaning that it concerns what SHOULD be done rather than what is, was, or could be. Normative work is elegant and enjoyable, but it often misses the messy scene that it would intervene into.[9] Human judgement is the final arbiter of political truth. The earth is getting warmer. It is likely that this will cause problems. These are facts. What is tricky is how these facts of techno-science are translated into a meaningful policy. Is the solution to outlaw cars or airplanes? Should all electrical generation be nuclear? Are hydroelectric dams (a major source of power at my current location) on balance better than coal?
Idealistic students are often vexed by the inability of the public to understand “science.” As Damien Pfister argues, the issue is not that the science of warming is in particular dispute, but that the translation of that into a viable policy is not a matter of techno-science but a question of policy, and the hockey stick graphic became a point where science, expertise, and participation interweaved.[10] Scientific authority is constructed around the discourse of description, the scientist’s instruments tell it how it is. The problem is that a measurement of atmospheric pressure is not particularly interesting. Among the most profound facts in our world are social facts – these include things like the unemployment rate and public opinion. These are deeply imperfect facts where the means of measurement were designed for the resolution of a problem, not the seeming objective question of science.
This is not to say that warming isn’t real, but that debates about warming are discussions of policy, not statements of fact.
4.1.6 Legal
Legal reasoning then is the application of an interpretation of what the law is to a particular set of facts. The framework for interpretation is largely determined by professional jurists, while the facts are determined by the rhetorical process of the jury trial. Juries serve as fact-finders. Once the jury determines what the facts are, courts up the chain of review operate on the basis of those facts when making their interpretations. Courts operating above the trial level, or appeals courts, are considering questions of interpretative theory.
The law itself is a combination of the laws passed by legislatures (like Congress), orders by executives (like the President), and regulations by agencies (like the FCC). These are then situated within the Constitutional framework that produced those laws and the tradition of common law. Common law principles provide a number of ideas in general operation, for example the common law would say that no one may be their own judge. In a country that recognizes the common law, the President would not be able to pardon himself as such a function of judgement cannot be done by alone.
Precedent is set by the Supreme Court of the United States (SCOTUS). They typically hear about ninety appeals each year. Cases are selected by the justices, this is called a writ of certiorari. The court only evaluates questions in the cases before it and the court is only the original jurisdiction for a handful of cases involving particularly thorny matters between state governments.[11] When we think of this in the context of the design of state institutions or speculative civics, the Courts in this sense are a trailing institution. They wait to take action.
Within the courts, precedent shapes the interpretation of what the law is. Precedents bind vertically, meaning that a court above makes a determination that should be followed below. This is called Stare Decisis.
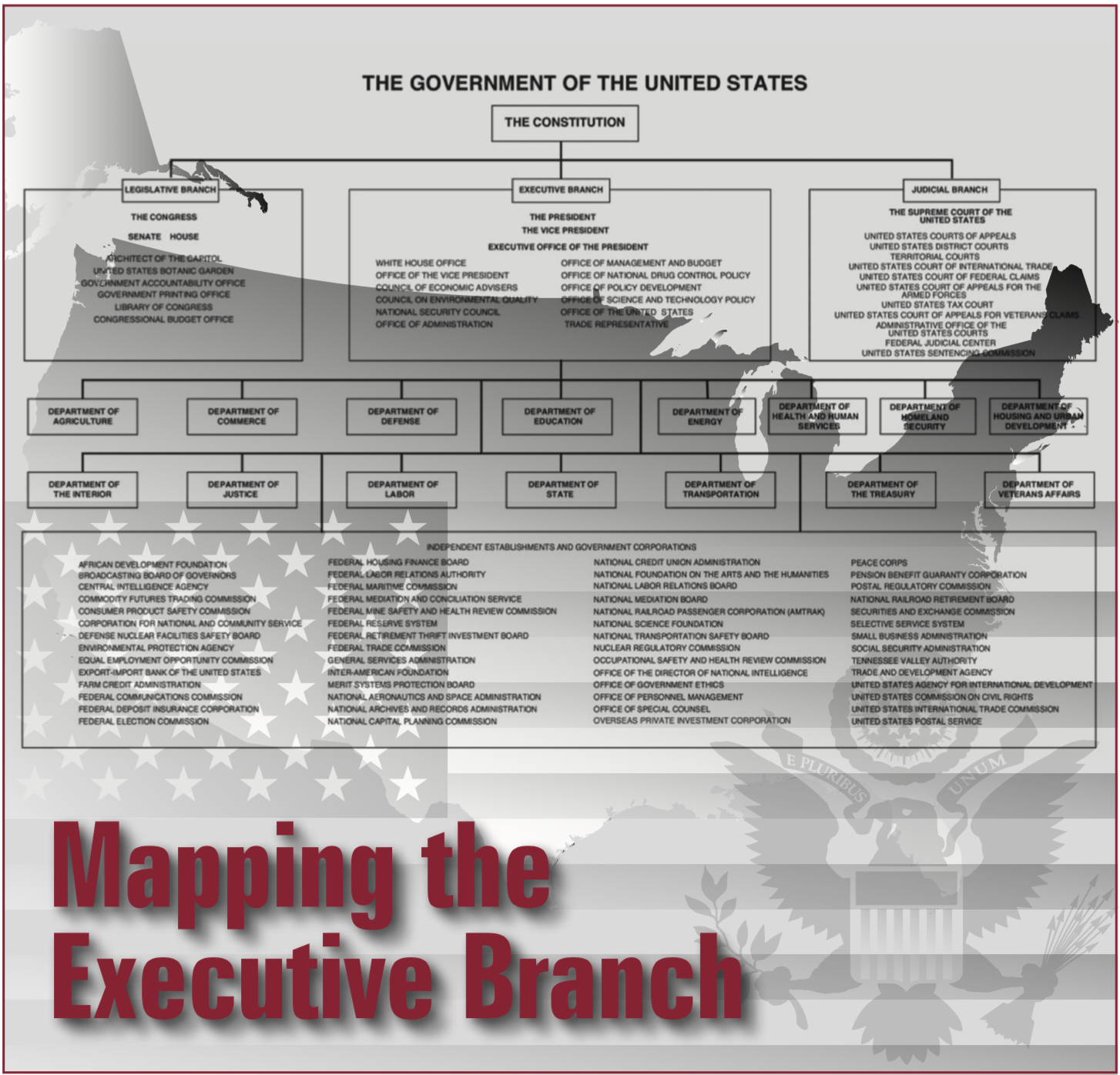
Generally, the Supreme Court is unlikely to overturn an existing precedent, opting instead to distinguish the current situation from that which formed the precedent in the first place. The law as such is the current interpretation of text and precedent. Black and Spriggs have found that depreciation occurs within a twenty-year window, it is not a question of all meaning over time, but how the courts have ruled recently.[12] If a case is not cited within the window, it is not likely that it will be included in the current understanding of the law. It is important to find a lawyer who is active in a domain of practice to understand what the law is actually understood to be in any particular area. A tax attorney may not be up to speed about current cable franchise law, and in areas where the court has been inactive in recent years, results may be far less predictable if half the justices on the court have never ruled in an area. Lawyers use many resources, including reference books like the restatement of law for a particular area to understand what the law actually is.
Why isn’t there a single answer or book with clear directions? There are many situations and different sets of facts. Much like the inability to directly translate the measurements of scientific devices into a policy finding, the analysis of the desirability of a result is very difficult to translate into an abstract legal rule.
Legal research is much like policy research, but tied more to a handful of hermeneutic frameworks. A central tenant of critical legal studies, a sub-discipline appreciated by communication researchers, holds that the law itself is indeterminate, that the text can be interpreted to have a wide variety of meanings. This is not to say that the legal text has no meaning, but that if there is an interpretive question it is more likely that the question will be resolved in favor of the side with more power.
4.1.6.1 Freedom of Expression
The First Amendment to the United States Constitution reads:
Congress shall make no law respecting an establishment of religion, or prohibiting the free exercise thereof; or abridging the freedom of speech, or of the press; or the right of the people peaceably to assemble, and to petition the Government for a redress of grievances.
This provides a right for Americans to distribute information, practice religion, and organize movements. If you have been doing your reading, you likely know many reasons why this is good. At the same time, you notice that this does not apply to non-state actors. If anything, private organizations have a first amendment right, in the context of the freedom of the press, to make editorial choices. It is not that we have a clear imperative to speak more, but that communication researchers are very interested in the careful balancing of public and private restraint and editorial judgement.
You may notice that this is a fairly limited right, it does not protect your right to say whatever you want without consequence, prohibit compelled speech, or state speech. There are powerful questions here:
- Should we protect lies?
- What do we do about hurtful speech?
- Should it be acceptable to say true things, even if they are hurtful?
- Does the truth actually win in public debate?
- How does speech intersect with intellectual property?
- What is journalism and should it have special protection?
- How should states respect the expression laws of other states?
- Should well-meaning restraints on communication be struck down if they both stop bad communication and chill good communication?
Freedom of expression is a major topic in communication and is a major theme for the future.
4.1.6.2 Intellectual Property
At this institution, Oregon State, IP law is covered extensively in Introduction to New Media. Intellectual property law includes:
Area | Topics | Protection |
Copyright | The protection of texts and authorship | Life of the author + 70 years; statutory protection for all registered texts ($250,000 per violation) |
Patent | Inventions that are useful, non-obvious, and correctly filed | Injunction, Damages |
Trademark | Derived from the Common Law, protection for the uniqueness of a brand or mark. Non-exclusive. | Injunction, Damages |
Trade Secret | Protection against theft of confidential information; criminal law | Criminal penalties and action to redress theft |
These regimes of law have distinct sources. Copyright and patent law are derived from article one section eight of the US Constitution. Trademark is a common-law protection. Trade secret is primarily a matter of uniform state law with new Federal law, and is primarily criminal law related to theft.
To be actionable under copyright law one must have made a derivative work for more than a transitory period of time. This means that parts of another work could be included in your work, and despite the originality of your new work you could be sued. The defense in this case is called “fair use” which supposes that there are legitimate uses of copyrighted material such as parody. There is no fair use for patented subject matter. Known as the patent bargain, the idea is that the invention is disclosed for the continuation of the process of science and improvement. Thus, new inventions that include another must pay royalties during the twenty-year period. In some cases, the period may be extended to make invention in a critical area more rewarding.
Patents were common in our area until the decision in CLS v. Alice – where the Supreme Court roundly rejected patents for applications that attempted to computerize existing business processes. It is unlikely that you will see business method patents in our area again. At the same time, you are likely so see patents protecting many technologies that we love. Without artificial scarcity, prices in this sector would drop below viable levels.
4.1.6.3 Open-Source
Many software libraries are open-source, meaning that they are provided to freely use and distribute. These resources like development platforms: Lens Studio, Android Studio, Xcode, Rstudio allow us a great deal of flexibility in making things. Proprietary API systems and the law of the API are likely major factors in our future. The underlying legal structure, at the point of this writing the Computer Abuse and Fraud Act of 1986, is quite unclear. This will be a major area to watch in the future, access controls provide a powerful and dangerous form of private law.
4.1.6.4 International
Data sovereignty is a very hot area right now. Countries around the globe are enacting laws regulating how information is managed in their jurisdiction, with Australia going as far as to require extensive backdoors into systems. There are extensive discussions in Global Media that were seemingly resolved by the techno-utopian vision of the internet that are clearly still very active. A deep understanding of the major frameworks for international relations: realism, neo-realism, liberalism, democratic peace theory, and constructivism will be critical going forward.
4.2 Quantitative
Hadley Wickham and Garrett Grolemund open their handy book R for Data Science, with an important distinction between hypothesis generating and hypothesis confirming quantitative research.[13] For the most part you are likely used to hypothesis testing as the horizon of quantitative research. The elision between quantitative methods and positivism can obscure the potential of other modes of assembling ideas. Hypothesis generating social science can use mathematical techniques and visualization to promote the creation of new interesting questions. In this section I am interested in discussing the foundations of a number of quantitative methods and how they contribute to media studies.
4.2.1 Discrete vs Continuous
Discrete implies that something is a single category. JL Austin’s classic example from speech act theory: one is either married or not married. There is no “sort of” married. There are thus two output variables. Continuous measures have a range of possible outputs, like temperatures. It is possible to render continuous as discrete through binning. We might say temperatures >50 and <50. Now if 50 is a meaningful number, describing a change in state, temperature could become discrete. Boiling versus non-boiling for instance is a meaningful discrete category.
4.2.2 Mathematical Moments
The central processes by which we consider the moments are: central tendency, variance, skewness, and kurtosis.[14] First, we are concerned with the center of the distribution, this can be found with mean or median. Mode is the most common value.
Variance is described by standard deviation of any individual data point from the mean. This is most commonly presented as standard deviation. If we consider a plot of a distribution, the skewness shows how the plot leans, and the kurtosis how sharp the peaks of the distribution.
These ideas allow you to think carefully about where measurement of a thing or a probability. Most of our statistical methods work with manipulations of measurements of central tendency and variance.
4.2.3 Hypothesis Testing
Hypothesis testing is an intrinsically digital way of knowing – it relies on the creation of an if-then construction which can be tested against a reference value. Confusion often comes in the discussion of what is truly tested, meaning the null hypothesis rather than the hypothesis itself. Hypothesis testing research seeks to confirm that something happened, thus rejecting the null hypothesis. Do we necessarily know what happened? No.
The ideas of type one and two error come from a foundational 1928 paper by Jezey Neyman and Egon Pearson:[15]
Accept Null | Reject Null | |
Null Accurate | Correct – nothing for nothing | Type 1: False Positive |
Non-Null Accurate | Type 2: False Negative | Correct – Something for Something |
The recurring remark is that there must also be a type three error related to answering the wrong question with the right method. In designing a study to use traditional hypothesis methods we consider the validity and reliability of the tests intended for the hypothesis.
Reliability means: do we get the same result twice when testing the same sample? Would you trust a glucometer that returned very different blood sugar readings on the same vial of blood? The reliability and margin of error for a test is important in designing an experiment. Studies are only as strong as their least reliable method.
Validity refers to the idea that the test in question actually answers the research question. These are divided into internal and external validity. Internal validity is the coherence of the design of the original logical statement, if X then Y. As you remember from the discussion of logical operators and transistors, these processes go in order and only one way. External validity is the ability to see that the result makes sense in the world, that it can be generalized. If a study of 300 undergraduates at a large state university who claim to have never heard of the rapper Drake (one of the most popular figures in popular music at this writing) crosses your desk you know that either A. the data are not legitimate or B. the undergraduates in the study are a highly atypical population and any extrapolation from them is risky at best.
There is one more substantial hurdle: the study needs to be physically possible. Consider the study of food and nutrition. To be ethical, a study must be beneficent, meaning that no one is harmed. It would be unethical to do research that involved intentionally starving people to see what vitamin does. Many social research questions may require surveys that exceed the capacity of the researcher or their funds. Research involving social network data is limited to the data that you can actually extract from that network, Facebook is not cooperative. There are many questions that are not practical to answer.
Consider the following study: This study is intended for 15 year olds. Question one: how many minutes of drinking have you seen in the last year on television? Question two: how many drinks do you have per day?
What do you imagine the results would be? Would you be comfortable with this use of self-report data? If the correlation was positive and the null hypothesis was rejected, would it be prudent to ban representations of beer drinking on television? Should you believe the recollections of the fifteen year olds over the last year or their current use reports?
Correlation, even if you assume the study is sound, does not imply causation. Although only a very silly person would reject the correlation of being hit by a bus and grievous physical injury.
Correlations are generally reported from -1 to 1 (meaning the slope line and relative noisiness) with zero being no relationship. Different coefficients have different properties. There are many other tests including T-Tests that compare the differences between populations. For example: if two comparable populations were exposed to some treatment and then asked for attitude change, the analysis of the variance within and between groups would be a useful measurement. These methods are not a perfect truth machine, they offer important information about central tendency and effect size. The key is understanding the limits of the tests in question and how they align with your research.
4.2.4 Iteration
It is unlikely that a social scientist will dream up the exact single experiment that could make sense of reality. The universe is simply too weird for that. We can expect many studies to be developed that circle in on a possible causal relationship that makes sense of the world. Keep in mind, that this process never ends. Methods become more reliable, our analysis of validity more-fine grained.
As we circle in on the hypothesis confirmation step, it is likely that the visualizations and thought processes will generate even more new hypotheses. As you may have notified in the discussion of policy research there is no quantitative translational moment. Good science is autotelic – it produces more of itself.
What is required is a sense of meta-awareness of what the field has done before. This is why the literature review section of a paper is so important, researchers need to know which hypotheses have already been rejected. This is also why replication is necessary, there need to be important checks both on hypotheses that seem to be true and those that are rejected as false. Most of all, science isn’t magic.
4.2.5 Phacking
The underlying criterion of many of these studies involves the use of the threshold for significance, which in social science is .05 which refers to the likelihood that the null would be rejected in error. The default condition is to reject the null if the level exceeds .05. Phacking refers to the intentional manipulation of a study to arrive at the .05 threshold, which seems both possible and suspiciously likely as so many studies tend to cluster at the key level.[16] Christine Ashwanden argues the problem is not necessarily that there is a great deal of cheating, but that doing really good research is really, really hard:
People often joke about the herky-jerky nature of science and health headlines in the media — coffee is good for you one day, bad the next — but that back and forth embodies exactly what the scientific process is all about. It’s hard to measure the impact of diet on health, Nosek told me. “That variation [in results] occurs because science is hard.” Isolating how coffee affects health requires lots of studies and lots of evidence, and only over time and in the course of many, many studies does the evidence start to narrow to a conclusion that’s defensible. “The variation in findings should not be seen as a threat,” Nosek said. “It means that scientists are working on a hard problem.”[17]
The popular rhetoric of science does little to help in this case. Science is presented as magical and somehow value free offering simple answers to political and ethical problems. In reality, science, like publicity, is a process. Easy answers are not coming.
Hypothesizing after the fact is another version of this problem where many measures are deployed and after some significant result is found a study is reconstructed around it. We should be careful not to delegitimize inductive qualitative strategies. Someone searching for a foothold might test thirty hypotheses. They would then report them and iterate the positive findings to generate more results. Perfection only matters for those with the least at stake. Exploratory data science is important for future hypothesis generation, at the same time exploratory work should not be passed off as something it isn’t.
When we consider the rhetoric of statistical design, there is a discourse that supposes that the double-blind controlled experiment is the only way to access the truth. If we reduce what we can know to only be that which is tested in this particular manner with a null rejection, there will be no knowledge left. Authority comes in the debunking of what would be meaningful results just as the skeptical game of hermeneutics becomes self-defeating when it hyper-signifies, statistics becomes decadent when the empirical is lost to the purely quantitative.
4.2.6 Bayesian, effect sizing, ANOVA, multiple hypothesis
For the purposes of this paragraph, you do not need to pretend that you care about football, but you need some awareness of what it is. How do we determine the best college football team in the country? Do we simply count wins and losses? There will be many teams with many wins, are we sure that the farm lads of Iowa play the same level competition as the engineers of MIT? Perhaps we should simply ask some keen sportsball fans? There are no easy answers.
Rankings are hard, especially when there are many teams (around 120) and only ten or eleven games per season. What do you do as the season progresses, how quickly should rankings change from week to week?
In the context of chess competition, a potential solution was proposed by Arpad Elo, which used a slow-moving evaluation of the quality of a player by adding the relative quality of other players to their ranking and dividing.[18] This is an important idea – we can assume that a player who has a very high ranking has likely beaten other very good players and lower ranked players have likely not won such difficult matches. Translating this back into the football example, we can assume that a 9-0 team from the University of Alabama (an institution known for superior football performance) would very likely defeat a team from Concordia College (a team known for its remarkable corncob mascot). Our assessment of Alabama anterior to the test (the game) would be that they were a very good football team, after the win (Concordia has no chance) it would not appreciably increase (we gained little new information). The ranking after would be the posterior measurement.
Instead of seeing the game as a chance to reject the hypothesis that Concordia is better at football than Alabama, the Bayesian method allows us to actually think about the level of information encoded in the game in an intuitive way. Bayesian methods are preferable as they are more easily sized to datasets, allow researchers to think about the world as it is (they are more empirical), and they are concerned with the analysis of variance and effect size. Playoff games would produce far more meaningful information as they involve seeded interactions between teams which we know to be excellent.
In his open letter calling for Bayesian methods in the psychological sciences, John Krushke make the following excellent points:
Some people may have the mistaken impression that the advantages of Bayesian methods are negated by the need to specify a prior distribution. In fact, the use of a prior is both appropriate for rational inference and advantageous in practical applications.
* It is inappropriate not to use a prior. Consider the well-known example of random disease screening. A person is selected at random to be tested for a rare disease. The test result is positive. What is the probability that the person actually has the disease? It turns out, even if the test is highly accurate, the posterior probability of actually having the disease is surprisingly small. Why? Because the prior probability of the disease was so small. Thus, incorporating the prior is crucial for coming to the right conclusion.
* Priors are explicitly specified and must be agreeable to a skeptical scientific audience. Priors are not capricious and cannot be covertly manipulated to predetermine a conclusion. If skeptics disagree with the specification of the prior, then the robustness of the conclusion can be explicitly examined by considering other reasonable priors. In most applications, with moderately large data sets and reasonably informed priors, the conclusions are quite robust.
* Priors are useful for cumulative scientific knowledge and for leveraging inference from small-sample research. As an empirical domain matures, more and more data accumulate regarding particular procedures and outcomes. The accumulated results can inform the priors of subsequent research, yielding greater precision and firmer conclusions.
* When different groups of scientists have differing priors, stemming from differing theories and empirical emphases, then Bayesian methods provide rational means for comparing the conclusions from the different priors.[19]
The advantages of working from a set of priors are clear: when you can debate the nature of the priors the underlying validity of the study can be determined in great detail. Rouder, Haaf, and Aust noted that Bayesian models are already becoming common in communication research.[20] Through a comparison of both approaches to a study of a story about refugees, they show that the null hypothesis would have rejected findings that could move the understanding of political communication forward. The sticking point would be the lack of a clear moment where the BayesFactor would call for the reporting of significance – the authors rightly critique such an assumption. The context of the discussion, more than some arbitrary number should drive the evaluation of the significance level.
Beyond Bayesian developments, methods like ANOVA which can deal with variance between multiple groups, structural equation modeling, and multiple hypothesis approaches are becoming more common. Even the basic techniques of social science are advancing, scientifically. Sometimes better science means a less convenient test of significance and a greater discussion of the qualitative.
4.2.7 The Replication Crisis
If Malcolm Gladwell has taught us anything, it is that counterintuitive results sell books. Among the fields that can produce the most fascinating counterintuitive results is social psychology, where seemingly small things are resented as having systemic effects on beliefs over a long period of time. One of the trickiest representational problems for media research is that many of the effects that we discuss should have some relatively simple experimental evidence.
One of the most commonly cited examples of the lack of reproducibility is priming theory.[21] Priming supposes that exposure to a word or image would unconsciously effect the cognition of a person afterward. For example, one of the most ridiculous examples is the idea that seeing a single image of an American flag can durably increase Republican voting intentions for months to come.[22]
Replication problems are endemic. Artificial intelligence researchers rarely share code or facilitate reproduction of their work.[23] Oncology papers had a 90% failure rate on replication.[24] In basic biological science, error rates for cell line identification are substantial.[25] What does that mean? When scientists apply a chemical to a sample of cells, they may not know what kind of cells they actually are.
Does this mean that science is bad or entirely fraudulent? No. It means that science is hard and the performance of credibility may often imbue unearned ethos for ostensibly scientific results. Researchers need to publish positive results that are interesting to continue their work. Aligning career results with experiment results is a short-circuit that will burn down the house of knowledge.
Some methods are not designed to produce identical results – network methods based on random walks vary based on the point at which the walk started. Unless random seeds in the methods are intentionally fixed, the graphic will not render the same way twice. Topic modeling systems will not assign the same topic number on multiple runs.
4.3 Big Data
MC Elish and danah boyd argue that the discourse of big data depends on “epistemological duct tape.”[26] The underlying methods of big data are quite routine, they are simply bigger. To hold things together, they identify the role of the rhetoric of magic – it becomes something of a strength as the model exists in a special place where normal rules don’t apply. The example of duct tape that is especially pressing is the idea of a “face detector,” as it is not a true detector of faces but a system that detects things that it was told fall into the category, faces.[27] Although this may seem like a trivial distinction it is really quite important as big data presents a difference in degree, not kind. In less abstract terms, if you had the wrong model on the small scale, getting bigger won’t make it right.
4.3.1 Resolving Assumptions
Given that most major models in big data are relatively straight forward, the major challenges in big data are in the area of validity. How do you resolve the collection of datasets of varying ages and qualities? If big data sets continue to affirm what we already know, is it better to made a decision without the cost and time of big data?
As you work with data science methods in communication, it will become clear that the fastest part of the process is executing the code. The much longer part of the process comes in cleaning and structuring the data and the code.
4.3.2 Inductive models
For the most part we begin with constructions that work abductively, we have probabilistic ideas about the truth and we work forward from there. What we can do with big data sets is run correlations and variance analyses on massive datasets. From these explorations, we might begin to see real power in running thousands of trials. These methods are implemented through neural networks, instead of looking for properties and directly testing, a neural network might use a chain of inductive cases to find an outcome. This outcome variable is not itself inductive – you selected by other means.
A key distinction to keep in mind is between supervised and unsupervised modeling. Supervised modeling retains regular human interaction, quality checking and maintaining the dataset on a regular basis. Unsupervised modeling would turn a process loose on a dataset without regular human intervention. There is still a real degree to which this is supervised in as much as the human operator selects which inputs to for the unsupervised process are present. One of the most important inductive moments today is the use of neural networks in unsupervised learning.
The first place you will see this as a student is in the selection of stopwords for natural language processing. In order to find more semantically rich terms, many articles and prepositions will be excluded from the dataset. This supposes that the terms that give sentences structure are unimportant, it seems possible that they are important as well, especially in ngrams. At the same time, as you tokenize and filter the data, you are losing information. If you have already taken the discussion of Bayes to heart, the use of stopwords will not be a huge problem for you. At the same time, you should feel a bit of trepidation as the level of interaction necessary to produce the results would seem to shift the study back toward the qualitative, you would be using less of that magic computational duct tape.
4.3.3 Direct Detection
The promise of big data for media research is direct detection of phenomena. Self-report data is notoriously unreliable. As one of my colleagues said to me on the street, “you know that anytime you ask an eighth grader if they do weird stuff, they are going to say yes – like lizard people.” This is an important problem. We can’t trust people to tell us real things about themselves. If you have time to work through the layers of fakeness and facework, ethnographic fieldwork can provide robust data with seemingly reversed data.
Social network scraping methods provide one sort of direct data. We can use APIs for the platforms to access Twitter and directly extract swarms of Tweets. Analysis of the Tweets themselves is possible. Unfortunately, this does not allow us to see how exactly the information diffused without response. In public sphere theory, the most common formulations of publics and counter-publics form by the attention of the audience rather than their participation.[28] Continuing with big data along these lines would require even more data about how systems work and some evidence that particular individuals saw something in the first place.
Other methods might involve securing sensor input data, such as information about the location of cell phones and their activity. Selfies could be reverse engineered to look for changes in the medical status of users. This is not the passive big data of magic, but a very active form of big data that calls for the active ingestion of massive datasets.
Self-reports and surveys are messy and inaccurate. Direct detection of the data offers a real transformation in social research. The challenge is getting access to directly detected data. Companies with such data are not often willing to share it, and publics increasingly wish to protect their data.
Topic modeling and sentiment analysis offer researchers the potential for working with directly detected data. Topic modeling produces a probabilistic model of the topics that should be assigned to particular documents within a corpus. This is limited by the relatively narrow confines of the interpretative frame detected. Sentiment analysis may be more limited, functioning primarily on the basis of join and count methods against existing dictionaries. At the same time, direct detection is promising as it offers new possibilities for the analysis of information.
Another form of direct detection comes in the form of bio-foundationalism. This could include the use of sensors, scanners, or other means to monitor the physical state of a person experiencing media. An EEG can allow detection of electrical activity in the brain through a skull cap, saliva samples can measure some chemical levels, blood is better in other cases. Deployed through less invasive methods an fMRI machine (which can detect the utilization of oxygen in the brain) or a PET scan (which detects the utilization of glucose) can give us indications about the use of faculties when exposed to certain kinds of media. These are direction detection methods and they offer a selection of answers to important questions but they are not themselves the entire answer to the problems posed by future communication.
4.4 Artificial Intelligence
Lt. Commander Data is a wonderful television character. As an emotionless, sentient android he offers a fresh take on the human condition. The promise of AI in this televisual sense provides a referent that is both profoundly human, creative, and more than human as a mechanical form. It is Data’s capacity for growth and adaptation that makes him a remarkable and is the magical promise of the system, much as the magic of big data is the prospect that we might ignore epistemology.
Much of what we call AI does not hinge on the development of a synthetic sentient form, but on raw processing power. Ian Bogost argues that the threshold to be called AI has dropped so far that simple string processing methods meet the term, meaning that your command or apple F in word is AI, as he put it:
By protecting the exalted status of its science-fictional orthodoxy, AI can remind creators and users of an essential truth: today’s computer systems are nothing special. They are apparatuses made by people, running software made by people, full of the feats and flaws of both.[29]
Computer vision technologies will allow more effective categorization of media products. Neural networks and other inductive methods will allow increasingly complex simulations of thought.
True artificial intelligence will be special and weird. It will not be quickly and easily added to a discussion board system.
4.5 Digital Humanities
The term digital humanities primarily refer to computationally enhanced literary studies. Consider a claim about the historical evolution of books, let’s say a book by Jimmy Jimmerson had a major impact on syntax as it moved across Italy. Instead of taking the word of scholars seriously that this book really was the hinge point, you can look at a comparison of the texts produced in the region during those times. If the style changes after the introduction of Jimmerson, you know that Jimmerson mattered. What if scholars made a claim that a certain film had an extremely balanced pattern of interaction among characters, would you take their word for it, or find a way to read the interactions in the next to produce a fingerprint of interaction? What would it mean for narrative scholarship if the ostensibly balanced story was, in fact, unbalanced?
What digital humanities typically means, is that contemporary quantitative tools are integrated into the quantification/descriptive side of a study. This does not mean that the qualitative interpretative or critical side of that study are eliminated. It is likely to be the reverse. Scholars now spend a great deal of time struggling with the layers between micro and macro, or invoking incomplete systems theories that skip levels. An economical computer model that can account for the selection of a key exemplar or provide a concise model of diffusion can make our research much more vivacious. This model of humanities scholarship has less hedging and qualification of claims, a better model for iteration of claims.
4.6 Visualization
A central concern for the future of research is the capacity to develop new graphics that can effectively communicate complex concepts. Narrative is underrated: good writing is an amazing vector for information transmission given the synthetic dimension of imagination and sensation. The technology of the novel is superior to the holodeck. Visualization can be misunderstood as the top of the continuum for digital humanities when there are really a number of important, next level, digital methods that intervene into debates and theories. To produce a visual, information must have been cleaned and organized. Visuals may include augmentations of reality, simulations, and abstract graphics. The simulations may be fruitful as they provide evidence of the structure of a system or they may provide this insight through the affective dimension of play.
Of the theorists of the rhetoric of the graphic, Edward Tufte is a clear leader. His theory of graphics rests on a seemingly linguistic break between “nouns” and “verbs” which is then framed by a procedure to reduce all graphics to their minimum necessary content.[30] The point of a great graphic is then to encode enough information for the viewer while maintaining a certain aesthetic sensibility. Some graphics intentionally over encode the information, becoming what Tufte refers to as “confections.”[31]
For the most part, efforts in contemporary information visualization do not pose new methods for graphical production, but increasingly smooth workflows. Data science coursework is really about the development of skills in R, Python, or another language that can facilitate these thoughts.
New immersive systems can provide complex models of the real physics of systems. These new models can provide real insight into the world in ways that were not possible without new displays and interfaces.
What is most important is that you understand that each of these new methods will have a grammar and a rhetoric. The best uses of these new tools will have a balance of aesthetic and conceptual information.
- C. Mantzavinos, “Hermeneutics,” in The Stanford Encyclopedia of Philosophy, ed. Edward N. Zalta, Winter 2016 (Metaphysics Research Lab, Stanford University, 2016), https://plato.stanford.edu/archives/win2016/entries/hermeneutics/. ↵
- Bryce, “There Is Nothing Outside of the Text,” Bryce E. Rich (blog), February 27, 2011, http://www.brycerich.com/2011/02/there-is-nothing-outside-of-the-text.html. ↵
- G.D. Robinsion, “Paul Ricoeur and the Hermeneutics of Suspicion: A Brief Overview and Critique,” Premise 2, no. 8 (September 27, 1995), http://individual.utoronto.ca/bmclean/hermeneutics/ricoeur_suppl/Ricoeur_Herm_of_Suspicion.htm. ↵
- Rita Felski, “Critique and the Hermeneutics of Suspicion,” M/C Journal 15, no. 1 (November 26, 2011), http://journal.media-culture.org.au/index.php/mcjournal/article/view/431. ↵
- Megan Geuss, “881 E.T. Cartridges Buried in New Mexico Desert Sell for $107,930.15,” Ars Technica, August 31, 2015, https://arstechnica.com/gaming/2015/08/881-e-t-cartridges-buried-in-new-mexico-desert-sell-for-107930-15/. ↵
- John Durham Peters, “Calendar, Clock, Tower” (Media in Transition 6, Massachusetts Institute of Technology, 2009), http://web.mit.edu/comm-forum/mit6/papers/peters.pdf. ↵
- John Durham Peters, Speaking into the Air: A History of the Idea of Communication (University of Chicago Press, 2001); Kittler, Fredrich, Gramaphone, Film, Typewriter, trans. Winthrop-Young, Geoffrey and Wutz, Michael (Stanford: Stanford University Press, 1999). ↵
- Richard Rumelt, Good Strategy, Bad Strategy (Currency, 2011), https://www.amazon.com/Good-Strategy-Bad-Difference-Matters/dp/0307886239. ↵
- Pierre Schlag, “Normative and Nowhere to Go,” Stanford Law Review 43, no. 1 (1990): 167–91. ↵
- Damien Smith Pfister, Networked Media, Networked Rhetorics: Attention and Deliberation in the Early Blogosphere (Penn State Press, 2014). ↵
- “Jurisdiction: Original, Supreme Court | Federal Judicial Center,” accessed November 20, 2018, https://www.fjc.gov/history/courts/jurisdiction-original-supreme-court. ↵
- Ryan C. Black and James F. II Spriggs, “The Citation and Depreciation of U.S. Supreme Court Precedent,” Journal of Empirical Legal Studies 10, no. 2 (2013): 325–58. ↵
- “R for Data Science,” accessed November 20, 2018, https://r4ds.had.co.nz/. ↵
- Eric W. Weisstein, “Moment,” Text, accessed November 20, 2018, http://mathworld.wolfram.com/Moment.html. ↵
- J. Neyman and E. S. Pearson, “On the Use and Interpretation of Certain Test Criteria for Purposes of Statistical Inference: Part I,” Biometrika 20A, no. 1/2 (1928): 177, https://doi.org/10.2307/2331945. ↵
- Christie Aschwanden, “Science Isn’t Broken,” FiveThirtyEight (blog), August 19, 2015, https://fivethirtyeight.com/features/science-isnt-broken/. ↵
- Ibid ↵
- His name is literally the name of the function. This is one of many implementations of the process. Nate Silver and Reuben Fischer-Baum, “How We Calculate NBA Elo Ratings,” FiveThirtyEight (blog), May 21, 2015, https://fivethirtyeight.com/features/how-we-calculate-nba-elo-ratings/. ↵
- John Krushke, “An Open Letter to Editors of Journals, Chairs of Departments, Directors of Funding Programs, Directors of Graduate Training, Reviewers of Grants and Manuscripts, Researchers, Teachers, and Students,” 2010, http://www.indiana.edu/~kruschke/AnOpenLetter.htm. ↵
- Jeffrey N. Rouder, Julia M. Haaf, and Frederik Aust, “From Theories to Models to Predictions: A Bayesian Model Comparison Approach,” Communication Monographs 85 (December 18, 2017): 41–56, https://doi.org/10.1080/03637751.2017.1394581. ↵
- Christine R. Harris et al., “Two Failures to Replicate High-Performance-Goal Priming Effects,” PLOS ONE 8, no. 8 (August 16, 2013): e72467, https://doi.org/10.1371/journal.pone.0072467. ↵
- Travis J. Carter, Melissa J. Ferguson, and Ran R. Hassin, “A Single Exposure to the American Flag Shifts Support toward Republicanism up to 8 Months Later,” Psychological Science 22, no. 8 (August 2011): 1011–18, https://doi.org/10.1177/0956797611414726. ↵
- Matthew Hutson, “Artificial Intelligence Faces Reproducibility Crisis,” Science 359, no. 6377 (February 16, 2018): 725–26, https://doi.org/10.1126/science.359.6377.725. ↵
- C. Glenn Begley, “Reproducibility: Six Red Flags for Suspect Work,” Nature 497 (May 22, 2013): 433–34, https://doi.org/10.1038/497433a.b ↵
- JH Duhnam and P Guthmiller, “Doing Good Science: Authenticating Cell Line Identity,” Corporate Page, Doing Good Science: Authenticating Cell Line Identity, 2012, https://www.promega.com/resources/pubhub/cell-line-authentication-with-strs-2012-update/. ↵
- M. C. Elish and danah boyd, “Situating Methods in the Magic of Big Data and AI,” Communication Monographs 85, no. 1 (January 2, 2018): 57–80, https://doi.org/10.1080/03637751.2017.1375130. ↵
- Ibid, 71. ↵
- Michael, Warner, “Publics and Counter-Publics,” Quarterly Journal of Speech 88 (2002): 413–25. ↵
- Ian Bogost, “‘Artificial Intelligence’ Has Become Meaningless,” The Atlantic, March 4, 2017, https://www.theatlantic.com/technology/archive/2017/03/what-is-artificial-intelligence/518547/. ↵
- E. R. Tufte, Envisioning Information. (Graphics Press, 1990), http://psycnet.apa.org/psycinfo/1990-97726-000. ↵
- Edward R Tufte, Visual Explanations: Images and Quantities, Evidence and Narrative (Cheshire, Conn.: Graphics Press, 2010). ↵